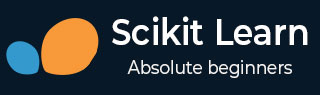
- Scikit 学习教程
- Scikit Learn - 主页
- Scikit Learn - 简介
- Scikit Learn - 建模过程
- Scikit Learn - 数据表示
- Scikit Learn - 估计器 API
- Scikit Learn - 约定
- Scikit Learn - 线性建模
- Scikit Learn - 扩展线性建模
- 随机梯度下降
- Scikit Learn - 支持向量机
- Scikit Learn - 异常检测
- Scikit Learn - K 最近邻
- Scikit Learn - KNN 学习
- 使用朴素贝叶斯分类
- Scikit Learn - 决策树
- 随机决策树
- Scikit Learn - Boosting 方法
- Scikit Learn - 聚类方法
- 集群性能评估
- 使用 PCA 降维
- Scikit Learn 有用资源
- Scikit Learn - 快速指南
- Scikit Learn - 有用的资源
- Scikit Learn - 讨论
Scikit Learn - 建模过程
本章介绍 Sklearn 中涉及的建模过程。让我们详细了解这一点并从数据集加载开始。
数据集加载
数据的集合称为数据集。它具有以下两个组成部分 -
特征- 数据的变量称为其特征。它们也称为预测变量、输入或属性。
特征矩阵- 它是特征的集合,以防有多个特征。
功能名称- 这是所有功能名称的列表。
响应- 它是基本上取决于特征变量的输出变量。它们也称为目标、标签或输出。
响应向量- 用于表示响应列。一般来说,我们只有一个回复栏。
目标名称- 它表示响应向量可能采用的值。
Scikit-learn 几乎没有示例数据集,例如用于分类的虹膜和数字以及用于回归的波士顿房价。
例子
以下是加载虹膜数据集的示例-
from sklearn.datasets import load_iris iris = load_iris() X = iris.data y = iris.target feature_names = iris.feature_names target_names = iris.target_names print("Feature names:", feature_names) print("Target names:", target_names) print("\nFirst 10 rows of X:\n", X[:10])
输出
Feature names: ['sepal length (cm)', 'sepal width (cm)', 'petal length (cm)', 'petal width (cm)'] Target names: ['setosa' 'versicolor' 'virginica'] First 10 rows of X: [ [5.1 3.5 1.4 0.2] [4.9 3. 1.4 0.2] [4.7 3.2 1.3 0.2] [4.6 3.1 1.5 0.2] [5. 3.6 1.4 0.2] [5.4 3.9 1.7 0.4] [4.6 3.4 1.4 0.3] [5. 3.4 1.5 0.2] [4.4 2.9 1.4 0.2] [4.9 3.1 1.5 0.1] ]
分割数据集
为了检查模型的准确性,我们可以将数据集分成两部分——训练集和测试集。使用训练集训练模型,使用测试集测试模型。之后,我们可以评估我们的模型的表现。
例子
下面的示例将数据按照70:30的比例进行分割,即70%的数据将用作训练数据,30%的数据将用作测试数据。数据集是 iris 数据集,如上例所示。
from sklearn.datasets import load_iris iris = load_iris() X = iris.data y = iris.target from sklearn.model_selection import train_test_split X_train, X_test, y_train, y_test = train_test_split( X, y, test_size = 0.3, random_state = 1 ) print(X_train.shape) print(X_test.shape) print(y_train.shape) print(y_test.shape)
输出
(105, 4) (45, 4) (105,) (45,)
如上例所示,它使用scikit-learn 的train_test_split()函数来分割数据集。该函数有以下参数 -
X, y - 这里,X是特征矩阵,y是响应向量,需要进行分割。
test_size - 这表示测试数据与总给定数据的比率。如上例所示,我们为 X 的 150 行设置test_data = 0.3。它将产生 150*0.3 = 45 行的测试数据。
random_size - 用于保证分割始终相同。这在您想要可重现结果的情况下非常有用。
训练模型
接下来,我们可以使用我们的数据集来训练一些预测模型。正如所讨论的,scikit-learn 拥有广泛的机器学习 (ML) 算法,这些算法具有一致的接口用于拟合、预测准确性、召回率等。
例子
在下面的示例中,我们将使用 KNN(K 最近邻)分类器。不要深入讨论 KNN 算法的细节,因为会有单独的章节来介绍。本示例仅用于让您了解实现部分。
from sklearn.datasets import load_iris iris = load_iris() X = iris.data y = iris.target from sklearn.model_selection import train_test_split X_train, X_test, y_train, y_test = train_test_split( X, y, test_size = 0.4, random_state=1 ) from sklearn.neighbors import KNeighborsClassifier from sklearn import metrics classifier_knn = KNeighborsClassifier(n_neighbors = 3) classifier_knn.fit(X_train, y_train) y_pred = classifier_knn.predict(X_test) # Finding accuracy by comparing actual response values(y_test)with predicted response value(y_pred) print("Accuracy:", metrics.accuracy_score(y_test, y_pred)) # Providing sample data and the model will make prediction out of that data sample = [[5, 5, 3, 2], [2, 4, 3, 5]] preds = classifier_knn.predict(sample) pred_species = [iris.target_names[p] for p in preds] print("Predictions:", pred_species)
输出
Accuracy: 0.9833333333333333 Predictions: ['versicolor', 'virginica']
模型持久化
训练模型后,最好保留该模型以供将来使用,这样我们就不需要一次又一次地重新训练它。这可以借助joblib包的转储和加载功能来完成。
考虑下面的示例,我们将保存上述训练模型(classifier_knn)以供将来使用 -
from sklearn.externals import joblib joblib.dump(classifier_knn, 'iris_classifier_knn.joblib')
上面的代码将模型保存到名为 iris_classifier_knn.joblib 的文件中。现在,可以借助以下代码从文件重新加载对象 -
joblib.load('iris_classifier_knn.joblib')
预处理数据
由于我们正在处理大量数据并且这些数据处于原始形式,因此在将数据输入机器学习算法之前,我们需要将其转换为有意义的数据。这个过程称为数据预处理。Scikit-learn为此提供了名为preprocessing 的包。预处理包具有以下技术 -
二值化
当我们需要将数值转换为布尔值时,会使用这种预处理技术。
例子
import numpy as np from sklearn import preprocessing Input_data = np.array( [2.1, -1.9, 5.5], [-1.5, 2.4, 3.5], [0.5, -7.9, 5.6], [5.9, 2.3, -5.8]] ) data_binarized = preprocessing.Binarizer(threshold=0.5).transform(input_data) print("\nBinarized data:\n", data_binarized)
在上面的例子中,我们使用阈值= 0.5,这就是为什么所有高于 0.5 的值都会转换为 1,所有低于 0.5 的值都会转换为 0。
输出
Binarized data: [ [ 1. 0. 1.] [ 0. 1. 1.] [ 0. 0. 1.] [ 1. 1. 0.] ]
均值去除
该技术用于消除特征向量的均值,使每个特征都以零为中心。
例子
import numpy as np from sklearn import preprocessing Input_data = np.array( [2.1, -1.9, 5.5], [-1.5, 2.4, 3.5], [0.5, -7.9, 5.6], [5.9, 2.3, -5.8]] ) #displaying the mean and the standard deviation of the input data print("Mean =", input_data.mean(axis=0)) print("Stddeviation = ", input_data.std(axis=0)) #Removing the mean and the standard deviation of the input data data_scaled = preprocessing.scale(input_data) print("Mean_removed =", data_scaled.mean(axis=0)) print("Stddeviation_removed =", data_scaled.std(axis=0))
输出
Mean = [ 1.75 -1.275 2.2 ] Stddeviation = [ 2.71431391 4.20022321 4.69414529] Mean_removed = [ 1.11022302e-16 0.00000000e+00 0.00000000e+00] Stddeviation_removed = [ 1. 1. 1.]
缩放
我们使用这种预处理技术来缩放特征向量。特征向量的缩放很重要,因为特征不应该综合地变大或变小。
例子
import numpy as np from sklearn import preprocessing Input_data = np.array( [ [2.1, -1.9, 5.5], [-1.5, 2.4, 3.5], [0.5, -7.9, 5.6], [5.9, 2.3, -5.8] ] ) data_scaler_minmax = preprocessing.MinMaxScaler(feature_range=(0,1)) data_scaled_minmax = data_scaler_minmax.fit_transform(input_data) print ("\nMin max scaled data:\n", data_scaled_minmax)
输出
Min max scaled data: [ [ 0.48648649 0.58252427 0.99122807] [ 0. 1. 0.81578947] [ 0.27027027 0. 1. ] [ 1. 0.99029126 0. ] ]
正常化
我们使用这种预处理技术来修改特征向量。特征向量的归一化是必要的,以便可以在公共尺度上测量特征向量。有两种类型的标准化,如下 -
L1 归一化
它也称为最小绝对偏差。它以这样的方式修改值,使得每行中的绝对值之和始终保持为 1。以下示例显示了对输入数据进行 L1 标准化的实现。
例子
import numpy as np from sklearn import preprocessing Input_data = np.array( [ [2.1, -1.9, 5.5], [-1.5, 2.4, 3.5], [0.5, -7.9, 5.6], [5.9, 2.3, -5.8] ] ) data_normalized_l1 = preprocessing.normalize(input_data, norm='l1') print("\nL1 normalized data:\n", data_normalized_l1)
输出
L1 normalized data: [ [ 0.22105263 -0.2 0.57894737] [-0.2027027 0.32432432 0.47297297] [ 0.03571429 -0.56428571 0.4 ] [ 0.42142857 0.16428571 -0.41428571] ]
L2 归一化
也称为最小二乘法。它以每行的平方和始终保持为 1 的方式修改该值。以下示例显示了对输入数据进行 L2 标准化的实现。
例子
import numpy as np from sklearn import preprocessing Input_data = np.array( [ [2.1, -1.9, 5.5], [-1.5, 2.4, 3.5], [0.5, -7.9, 5.6], [5.9, 2.3, -5.8] ] ) data_normalized_l2 = preprocessing.normalize(input_data, norm='l2') print("\nL1 normalized data:\n", data_normalized_l2)
输出
L2 normalized data: [ [ 0.33946114 -0.30713151 0.88906489] [-0.33325106 0.53320169 0.7775858 ] [ 0.05156558 -0.81473612 0.57753446] [ 0.68706914 0.26784051 -0.6754239 ] ]