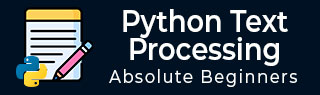
- Python-文本处理
- Python-文本处理简介
- Python - 文本处理环境
- Python - 字符串不变性
- Python - 排序行
- Python - 重新格式化段落
- Python - 计算段落中的标记
- Python - 二进制 ASCII 转换
- Python - 字符串作为文件
- Python-向后读取文件
- Python - 过滤重复单词
- Python - 从文本中提取电子邮件
- Python - 从文本中提取 URL
- Python - 漂亮的打印
- Python - 文本处理状态机
- Python - 大写和翻译
- Python - 标记化
- Python - 删除停用词
- Python - 同义词和反义词
- Python - 文本翻译
- Python-单词替换
- Python-拼写检查
- Python - WordNet 接口
- Python - 语料库访问
- Python - 标记单词
- Python - 块和缝隙
- Python - 块分类
- Python-文本分类
- Python-二元组
- Python - 处理 PDF
- Python-处理Word文档
- Python - 读取 RSS 提要
- Python-情感分析
- Python - 搜索和匹配
- Python - 文本修改
- Python-文本换行
- Python-频率分布
- Python-文本摘要
- Python - 词干算法
- Python - 约束搜索
Python-频率分布
在文本处理过程中,经常需要统计某个词在文本正文中出现的频率。这可以通过应用word_tokenize()函数并将结果附加到列表中以保持单词计数来实现,如下面的程序所示。
from nltk.tokenize import word_tokenize from nltk.corpus import gutenberg sample = gutenberg.raw("blake-poems.txt") token = word_tokenize(sample) wlist = [] for i in range(50): wlist.append(token[i]) wordfreq = [wlist.count(w) for w in wlist] print("Pairs\n" + str(zip(token, wordfreq)))
当我们运行上面的程序时,我们得到以下输出 -
[([', 1), (Poems', 1), (by', 1), (William', 1), (Blake', 1), (1789', 1), (]', 1), (SONGS', 2), (OF', 3), (INNOCENCE', 2), (AND', 1), (OF', 3), (EXPERIENCE', 1), (and', 1), (THE', 1), (BOOK', 1), (of', 2), (THEL', 1), (SONGS', 2), (OF', 3), (INNOCENCE', 2), (INTRODUCTION', 1), (Piping', 2), (down', 1), (the', 1), (valleys', 1), (wild', 1), (,', 3), (Piping', 2), (songs', 1), (of', 2), (pleasant', 1), (glee', 1), (,', 3), (On', 1), (a', 2), (cloud', 1), (I', 1), (saw', 1), (a', 2), (child', 1), (,', 3), (And', 1), (he', 1), (laughing', 1), (said', 1), (to', 1), (me', 1), (:', 1), (``', 1)]
条件频率分布
当我们想要计算满足一组文本的特定条件的单词时,使用条件频率分布。
import nltk #from nltk.tokenize import word_tokenize from nltk.corpus import brown cfd = nltk.ConditionalFreqDist( (genre, word) for genre in brown.categories() for word in brown.words(categories=genre)) categories = ['hobbies', 'romance','humor'] searchwords = [ 'may', 'might', 'must', 'will'] cfd.tabulate(conditions=categories, samples=searchwords)
当我们运行上面的程序时,我们得到以下输出 -
may might must will hobbies 131 22 83 264 romance 11 51 45 43 humor 8 8 9 13